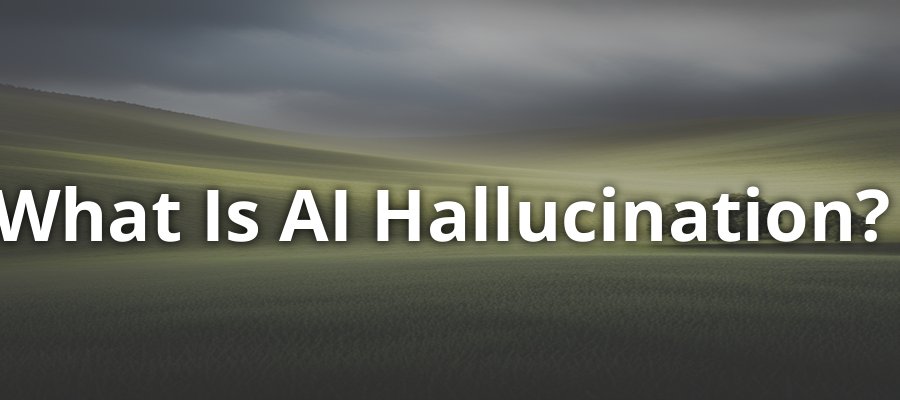
Understanding AI Hallucination
Artificial Intelligence (AI) has made significant strides in various fields, offering solutions that were once considered the realm of science fiction. However, as these technologies advance, they also encounter unique challenges. One such challenge is AI hallucination, a phenomenon that can lead to misinformation, misinterpretation, and unintended consequences in AI applications. This section delves into the concept of AI hallucination, providing a comprehensive understanding of its definition, examples, types, and causes.
1.1 Defining AI Hallucination
AI hallucination refers to instances where generative AI models, such as large language models (LLMs) or computer vision systems, produce outputs that are not grounded in reality or the data they were trained on. These outputs can range from slightly inaccurate interpretations to completely nonsensical or fabricated information. Unlike human hallucinations, which are perceptual experiences not based on external stimuli, AI hallucinations arise from the model's internal processes and its interaction with the training data.
The phenomenon is particularly prevalent in models that generate text or images, where the complexity of understanding and replicating human language or visual perception leads to errors. AI hallucinations are not merely technical glitches but are indicative of deeper issues related to how AI models understand and process information.
1.2 Examples and Types of AI Hallucinations
AI hallucinations manifest in various forms across different AI applications. One notable example is in natural language processing (NLP), where a chatbot might generate a plausible but entirely fictional story or fact in response to a user's query. In computer vision, an AI might 'see' objects in an image that are not present, similar to how humans might perceive shapes in clouds.
These hallucinations can be categorized into two main types: benign and malicious. Benign hallucinations, though incorrect, do not cause harm or spread misinformation intentionally. They often result from the model's overfitting or lack of sufficient training data. Malicious hallucinations, on the other hand, can have serious implications, especially if the AI is used in critical applications like healthcare diagnosis, where false information could lead to incorrect treatments.
1.3 The Causes Behind AI Hallucinations
Several factors contribute to AI hallucinations, with the primary cause being the inherent limitations of the models themselves. AI models, especially generative ones, rely on patterns found in their training data to make predictions or generate outputs. When faced with inputs that are significantly different from their training data, or when the data itself contains biases or inaccuracies, the model may 'hallucinate' responses.
Overfitting is another significant cause, where the model learns the training data too well, including its noise and anomalies, leading to poor performance on new, unseen data. Additionally, the complexity of the model and the algorithms used can also contribute to hallucinations, as more complex models are harder to interpret and control.
Understanding the causes and manifestations of AI hallucinations is crucial for developing strategies to mitigate their impact, ensuring that AI technologies remain reliable and beneficial across various applications.
Addressing AI Hallucination
AI hallucination poses significant challenges in the deployment and trustworthiness of AI systems. Addressing these challenges requires a multifaceted approach, focusing on prevention strategies and detection methods. This section delves into the techniques and tools available to mitigate the impact of AI hallucinations, ensuring AI systems function as intended and maintain user trust.
Strategies to Prevent AI Hallucinations
Preventing AI hallucinations is paramount to maintaining the integrity and reliability of AI systems. The following strategies are essential in minimizing the occurrence of hallucinations in AI models.
Use High-Quality Training Data
The foundation of any AI model is its training data. High-quality, diverse, balanced, and well-structured data sets are crucial in preventing AI hallucinations. Ensuring the data accurately represents the problem space minimizes output bias and improves the model's understanding of its tasks, leading to more effective and accurate outputs.
Define the Purpose of Your AI Model
Clearly defining the purpose and limitations of an AI model helps in reducing hallucinations. Establishing the system’s responsibilities and limitations guides the AI in completing tasks more effectively, minimizing irrelevant or hallucinatory results.
Use Data Templates
Data templates provide a predefined format for AI models, increasing the likelihood of generating outputs that align with prescribed guidelines. This approach ensures output consistency and reduces the chances of producing erroneous results.
Limit Responses
Constraining AI models by defining boundaries and using filtering tools or probabilistic thresholds can significantly reduce hallucinations. Limiting possible outcomes improves the consistency and accuracy of the model's results.
Test and Refine Continually
Rigorous testing before deployment and ongoing evaluation are critical in preventing AI hallucinations. Continual testing and refinement allow for adjustments and retraining as necessary, improving the system's performance over time.
Rely on Human Oversight
Human oversight is a crucial backstop measure in preventing AI hallucinations. Validating and reviewing AI outputs by humans ensures that any hallucinatory outputs can be identified and corrected promptly.
Detecting AI Hallucinations: Methods and Tools
Detecting AI hallucinations is as crucial as preventing them. The following methods and tools are instrumental in identifying and addressing hallucinations in AI models.
Monitoring and Evaluation Tools
Implementing monitoring and evaluation tools that track the performance and outputs of AI models in real-time can help in detecting hallucinations. These tools can alert developers to anomalies or unexpected outputs, prompting further investigation.
Adversarial Testing
Adversarial testing involves challenging the AI model with inputs designed to test the limits of its understanding and output generation capabilities. This method helps in identifying vulnerabilities, including the propensity for hallucination.
Transparency and Explainability Tools
Tools that enhance the transparency and explainability of AI models can aid in detecting hallucinations. Understanding why a model generates a particular output can help in identifying when and why hallucinations occur.
Feedback Loops
Incorporating feedback loops where users can report unexpected or incorrect outputs can help in detecting hallucinations. User feedback is invaluable in identifying issues that may not be apparent during testing phases.
Collaborative Filtering
Collaborative filtering involves comparing outputs across multiple models or instances to identify outliers or hallucinations. This method leverages the wisdom of the crowd principle to detect and correct hallucinatory outputs.
In conclusion, addressing AI hallucination requires a comprehensive approach that includes prevention strategies and detection methods. By implementing these techniques and tools, developers can minimize the occurrence of hallucinations, ensuring AI systems are reliable, trustworthy, and effective in their intended applications.
AI Hallucination in Practice
AI hallucination, a phenomenon where AI systems generate outputs based on patterns or objects that do not exist, has significant implications for both business operations and ethical considerations. This section delves into the practical aspects of AI hallucination, exploring its impact on business and ethics, and projecting future trends and research directions in the field.
3.1 Implications for Business and Ethics
AI hallucination poses unique challenges and opportunities for businesses. On one hand, it can lead to innovative applications in creative industries, such as art and design, by generating novel and imaginative outputs. On the other hand, it can cause serious consequences in critical sectors like healthcare, where an incorrect diagnosis could lead to unnecessary or harmful medical interventions.
Ethical Considerations
The ethical implications of AI hallucination are profound. Misinformation generated by AI can spread rapidly, undermining public trust and safety. For instance, a news-generating AI that hallucinates could disseminate false information about a public health emergency, exacerbating the situation. Therefore, it is crucial for organizations to implement rigorous testing and validation processes to minimize the risk of hallucination.
Business Impact
From a business perspective, AI hallucination can affect decision-making processes, customer interactions, and brand reputation. Inaccurate data analysis or customer service responses can lead to misguided decisions, customer dissatisfaction, and damage to the company's reputation. Businesses must be vigilant in monitoring AI outputs and ready to intervene when inaccuracies are detected.
3.2 Future Trends and Research Directions
The ongoing evolution of AI technology suggests that hallucination will remain a critical area of focus. Research is increasingly directed towards understanding the underlying causes of AI hallucination and developing more robust models that are less prone to this phenomenon.
Advancements in AI Models
Future AI models are likely to incorporate advanced mechanisms for self-correction and validation, reducing the incidence of hallucination. Techniques such as adversarial training, where models are exposed to both normal and manipulated inputs, are expected to play a significant role in enhancing AI reliability.
Ethical AI Development
The ethical development of AI will gain prominence, with a focus on creating transparent, accountable, and fair AI systems. This includes developing guidelines for the ethical use of AI, ensuring diversity in training data, and involving human oversight in AI decision-making processes.
Industry-Specific Solutions
Research will also focus on developing industry-specific solutions to address the unique challenges posed by AI hallucination in different sectors. For example, in healthcare, AI models might be designed with additional safeguards and validation steps to ensure the accuracy of diagnoses and treatment recommendations.
In conclusion, AI hallucination is a complex phenomenon with significant implications for business and ethics. As AI technology continues to evolve, addressing the challenges of hallucination will require ongoing research, ethical considerations, and industry-specific solutions to harness the benefits of AI while minimizing its risks.